Data Sharing Models For State Longitudinal Data Systems
Jeremias Solari, Assistant Director
June 9, 2021
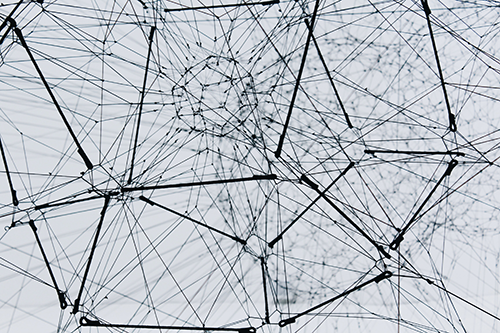
State Longitudinal Data Systems (“system” is an information technology system for the creation of longitudinal data) come in different “flavors” and differing levels of completion and complexity. Although there is a vast amount of diversity amongst the states’ systems, one criterion that defines systems into two major groups is their data sharing and governance model. Specifically, whether or not a given system is federated or centralized.
Federated or Centralized
In a federated model of data sharing, the data partners provide data in an ad hoc manner. For example, a request is received from a third party attempting to investigate the effects of specific course-taking pathways on ultimate higher education outcomes. The appropriate data partners would communicate data to the system; the system would match, de-identify, and provide the data to the requestor. At a prescribed time in the future, these data would be deleted from the system as the request is fulfilled and no longer necessary. In this model, the system acts as a clearinghouse, and the data have a life cycle that includes deletion; therefore, they are said to be non-persistent.
The opposite is the case with a centralized model. In this data-sharing model, the data partners periodically provide data to the system (e.g., monthly, quarterly, annually, etc.)—think of a data lake. These data are matched and de-identified, made available in a centralized repository for research, and are said to be persistent. In this model, the data exists regardless of requests or usage, and they embody the “longitudinal” system component.
There are pros and cons to both of these approaches. Below are some of the more meaningful:
- Data partners have more direct control over how data is used in a federated model
- The concept of longitudinal data is an actuality with a centralized model
- There are arguments for cost savings with both systems. This savings is very dependent on the scale of data requests. Centralized systems have the edge over federated if the scale of data requests is large, and vice versa if it is low
- Subject matter expertise of the longitudinal pipeline is potentially optimized in a centralized system
- The data structures in a centralized system may lead to higher data quality at the cost of speed
- The data structures in a federated system may lead to higher speeds at the expense of data quality
Regardless of the data-sharing model, there have been success stories for both of these modalities. At the core, systems built upon either paradigm have the same goal: making data available to leverage value. Lastly, we wanted to be specific in informing our readers that the UDRC is indeed a centralized system.